By harnessing artificial intelligence and modern computing, scientists are simulating more complex biological, clinical and public health phenomena to accelerate discovery.
While scientists have always employed a vast set of methods to observe the immense worlds among and beyond our solar system, in our planet’s many ecosystems, and within the biology of Earth’s inhabitants, the public’s perception tends to reduce this mosaic to a single portrait.
A Google image search will reaffirm that the classic image of the scientist remains a person in a white coat staring intently at a microscope or sample in a beaker or petri dish. Many biomedical researchers do still use their fair share of glassware and plates while running experiments. These scientists, however, now often need advanced computational techniques to analyze the results of their studies, expanding the array of tools researchers must master to push knowledge forward. For every scientist pictured pipetting, we should imagine others writing code or sending instructions to a supercomputer.
In some cases, scientists are testing whether computers can be used to simulate the experiments themselves. Computational tools such as generative artificial intelligence (AI) may be able to help scientists improve data inputs, create scenarios and generate synthetic data by simulating biological processes, clinical outcomes and public health campaigns. Advances in simulation one day might help scientists more quickly narrow in on promising results that can be confirmed more efficiently through real-world experiments.
“There are many different types of simulation in the life sciences,” says Kevin Yip, PhD, professor in the Cancer Genome and Epigenetics Program at Sanford Burnham Prebys and director of the Bioinformatics Shared Resource. “Molecular simulators, for example, have been used for a long time to show how certain molecules will change their shape and interact with other molecules.”
“One of the most successful examples is in structural biology with the program AlphaFold, which is used to predict protein structures and interactions,” adds Yip. “This program was built on a very solid foundation of actual experiments determining the structures of many proteins. This is something that other fields of science can work to emulate, but in most other cases simulation continues to be a work in progress rather than a trusted technique.”
In the Sanford Burnham Prebys Conrad Prebys Center for Chemical Genomics (Prebys Center), scientists are using simulation-based techniques to more effectively and efficiently find new potential drugs.
To expedite their drug discovery and optimization efforts, the Prebys Center team uses a suite of computing tools to run simulations that model the fit between proteins and potential drugs, how long it will take for drugs to break down in the body, and the likelihood of certain harmful side effects, among other properties.
“In my group, we know what the proteins of interest look like, so we can simulate how certain small molecules would fit into those proteins to try and design ones that fit really well,” says Steven Olson, PhD, executive director of Medicinal Chemistry at the Prebys Center. In addition to fit, Olson and team look for drugs that won’t be broken down too quickly after being taken.
“That can be the difference between a once-a-day drug and one you have to take multiple times a day, and we know that patients are less likely to take the optimal prescribed dose when it is more than once per day,” notes Olson.
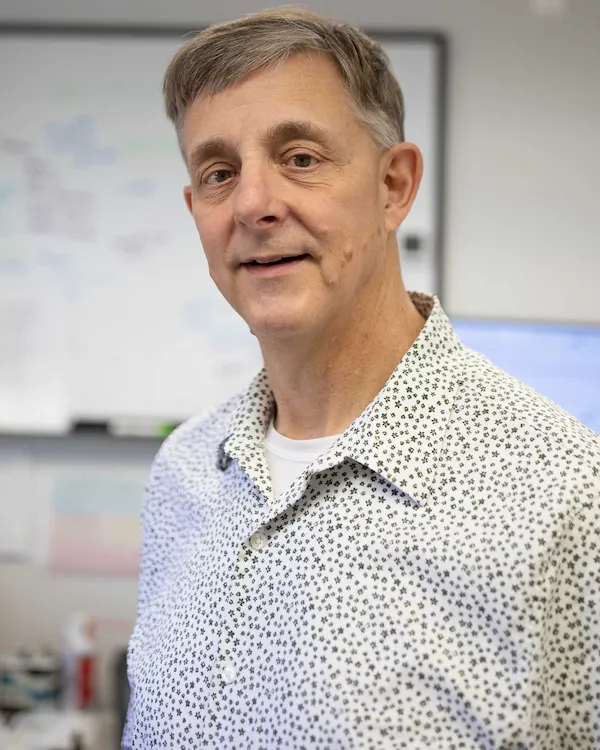
Steven Olson, PhD, is the executive director of Medicinal Chemistry at the Prebys Center.
“We can use computers now to design drugs that stick around and achieve concentrations that are pharmacologically effective and active. What the computers produce are just predictions that still need to be confirmed with actual experiments, but it is still incredibly useful.”
In one example, Olson is working with a neurobiologist at the University of California Santa Barbara and an x-ray crystallographer at the University of California San Diego on new potential drugs for Alzheimer’s disease and other forms of dementia.
“This protein called farnesyltransferase was a big target for cancer drug discovery in the 1990s,” explains Olson. “While targeting it never showed promise in cancer, my collaborator showed that a farnesyltransferase inhibitor stopped proteins from aggregating in the brains of mice and creating tangles, which are a pathological hallmark of Alzheimer’s.”
“We’re working together to make drugs that would be safe enough and penetrate far enough into the brain to be potentially used in human clinical trials. We’ve made really good progress and we’re excited about where we’re headed.”
To expedite their drug discovery and optimization efforts, Olson’s team uses a suite of computing tools to run simulations that model the fit between proteins and potential drugs, how long it will take for drugs to break down in the body, and the likelihood of certain harmful side effects, among other properties. The Molecular Operating Environment program is one commercially available application that enables the team to visualize candidate drugs’ 3D structures and simulate interactions with proteins. Olson and his collaborators can manipulate the models of their compounds even more directly in virtual reality by using another software application known as Nanome. DeepMirror is an AI tool that helps predict the potency of new drugs while screening for side effects, while StarDrop uses learning models to enable the team to design drugs that aren’t metabolized too quickly or too slowly.
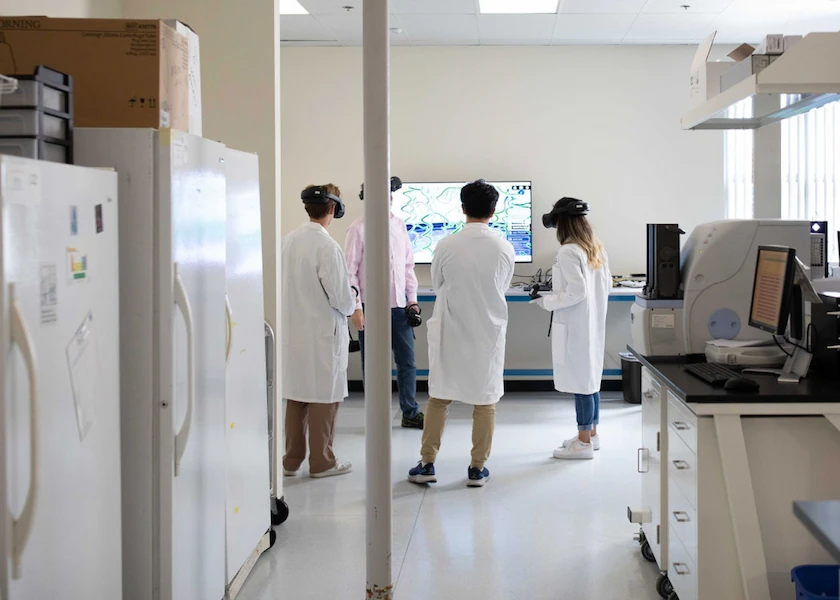
The Prebys Center team demonstrates how the software application known as Nanome allows scientists to manipulate the models of potential drug compounds directly in virtual reality.
“In addition, there are certain interactions that can only be understood by modeling with quantum mechanics,” Olson notes. “We use a program called Gaussian for that, and it is so computationally intense that we have to run it over the weekend and wait for the results.”
“We use these tools to help us visualize the drugs, make better plans and give us inspiration on what we should make. They also can help explain the results of our experiments. And as AI improves, it’s helping us to predict side effects, metabolism and all sorts of other properties that previously you would have to learn by trial and error.”
While simulation is playing an active and growing role in drug discovery, Olson continues to see it as complementary to the human expertise required to synthesize new drugs and put predictions to the test with actual experiments.
“The idea that we’re getting to a place where we can simulate the entire drug design process, that’s science fiction,” says Olson. “Things are evolving really fast right now, but I think in the future you’re still going to need a blend of human brainpower and computational brainpower to design drugs.”
Programming in a Petri Dish, an 8-part series
How artificial intelligence, machine learning and emerging computational technologies are changing biomedical research and the future of health care
- Part 1 – Using machines to personalize patient care. Artificial intelligence and other computational techniques are aiding scientists and physicians in their quest to prescribe or create treatments for individuals rather than populations.
- Part 2 – Objective omics. Although the hypothesis is a core concept in science, unbiased omics methods may reduce attachments to incorrect hypotheses that can reduce impartiality and slow progress.
- Part 3 – Coding clinic. Rapidly evolving computational tools may unlock vast archives of untapped clinical information—and help solve complex challenges confronting health care providers.
- Part 4 – Scripting their own futures. At Sanford Burnham Prebys Graduate School of Biomedical Sciences, students embrace computational methods to enhance their research careers.
- Part 5 – Dodging AI and computational biology dangers. Sanford Burnham Prebys scientists say that understanding the potential pitfalls of using AI and other computational tools to guide biomedical research helps maximize benefits while minimizing concerns.
- Part 6 – Mapping the human body to better treat disease. Scientists synthesize supersized sets of biological and clinical data to make discoveries and find promising treatments.
- Part 7 – Simulating science or science fiction? By harnessing artificial intelligence and modern computing, scientists are simulating more complex biological, clinical and public health phenomena to accelerate discovery.
- Part 8 – Acceleration by automation. Increases in the scale and pace of research and drug discovery are being made possible by robotic automation of time-consuming tasks that must be repeated with exhaustive exactness.