Artificial intelligence (AI) and other computational techniques are aiding scientists and physicians in their quest to create treatments for individuals rather than populations
The Human Genome Project captured the public’s imagination with its global quest to better understand the genetic blueprint stored on the DNA within our cells. The project succeeded in delivering the first-ever sequence of the human genome while foreshadowing a future for medicine once considered to be science fiction. The project presaged the possibility that health care could be personalized based on clues within a patient’s unique genetic code.
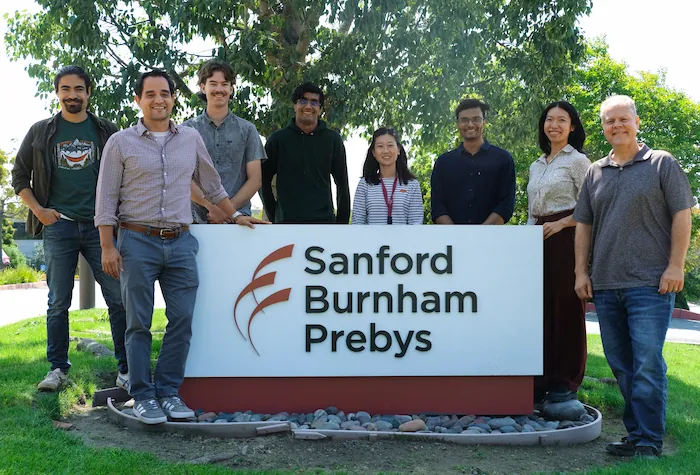
The Chavez Lab
While many more people have undergone genetic testing through consumer genealogy and health services such as 23andMe and Ancestry than through health care systems, genomic sequencing has influenced clinical care in some specialties. Personalized medicine—also known as precision medicine or genomic medicine—has been especially helpful for people suffering from rare diseases that historically have been difficult to diagnose and treat.
Scientists at Sanford Burnham Prebys are employing new technologies and expertise to test ways to improve diagnoses and customize treatments for many diseases based on unique characteristics within tumors, blood samples and other biopsies.
AI and other computational techniques are enabling patient samples to be rapidly analyzed and compared to data from vast numbers of individuals who have been treated for the same condition. Physicians can use AI and other tools to identify subtypes of cancers and other conditions, as well as improve selection of eligible candidates for clinical trials.
“I think we’ve gotten a lot better at precision diagnostics,” says Lukas Chavez, PhD, an assistant professor in the Cancer Genome and Epigenetics Program at Sanford Burnham Prebys. “In my work at Rady Children’s Hospital in cancer, we can characterize a tumor based on mutations, including predicting how quickly different tumors will spread. What we too often lack, however, are better treatment approaches or medicines. That will be the next generation of precision medicine.”
Sanju Sinha, PhD, an assistant professor in the Cancer Molecular Therapeutics Program at Sanford Burnham Prebys, is developing projects to help bridge the gap between precision diagnostics and treatment. He is partnering with the National Cancer Institute on a first-of-its-kind computational tool to systematically predict patient response to cancer drugs at single-cell resolution.
A study published in the journal Nature Cancer discussed how the tool, called PERCEPTION, was successfully validated by predicting the response to individual therapies and combination treatments in three independent published clinical trials for multiple myeloma, breast and lung cancer.
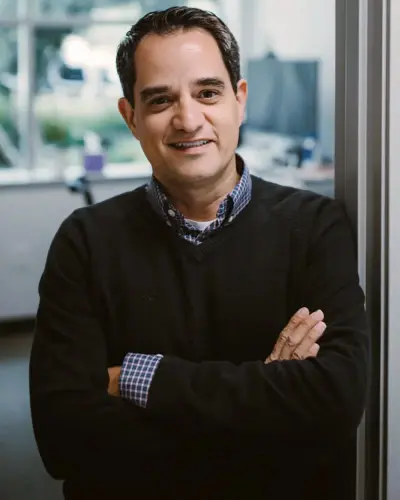
Lukas Chavez, PhD, is an assistant professor in the Cancer Genome and Epigenetics Program at Sanford Burnham Prebys.
In each case, PERCEPTION correctly stratified patients into responder and non-responder categories. In lung cancer, it even captured the development of drug resistance as the disease progressed, a notable discovery with great potential.
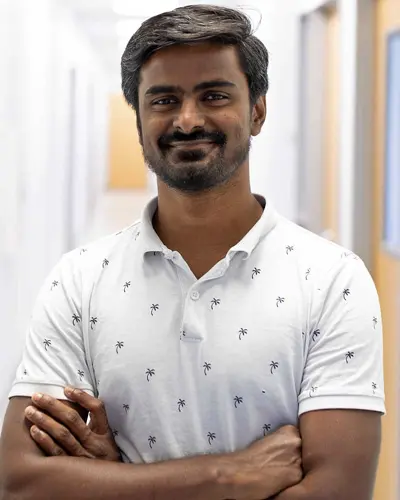
Sanju Sinha, PhD, is an assistant professor in the Cancer Molecular
Therapeutics Program at Sanford Burnham Prebys.
“The ability to monitor the emergence of resistance is the most exciting part for me,” says Sinha. “It has the potential to allow us to adapt to the evolution of cancer cells and even modify our treatment strategy.”
While PERCEPTION is not yet ready for clinics, Sinha hopes that widespread adoption of this technology will generate more data, which can be used to further develop and refine the technology for use by health care providers.
In another project, Sinha is focused on patients being treated for potential cancers that may never progress into dangerous conditions warranting treatment and its accompanying side effects.
“Many women who are diagnosed with precancerous changes in the breast seek early treatment,” says Sinha. “Most precancerous cells never lead to cancer, so it may be that as many as eight of 10 women with this diagnosis are being overtreated, which is a huge issue.”
To try and counter this phenomenon, Sinha is training AI models on images of biopsied samples in conjunction with multi-omics sequencing data. His team’s goal is to develop a tool capable of predicting which patients’ cancers would progress based on the imaged samples alone.
“In the field of precancer, insurance does not cover the cost of computing this omics data,” says Sinha. “Health care systems do routinely generate histopathological slides from patient biopsies, so we feel that a tool leveraging these images could be a scalable and accessible solution.”
If Sinha’s team is successful, an AI tool integrated into clinics would predict whether precancerous cells would progress within the next 10 years to guide treatment decisions and how patients are monitored.
“With precision medicine, our hope is not to just treat patients with better drugs, but also to make sure that patients are not unnecessarily treated and made to bear needless costs and side effects that disrupt their quality of life.”
Programming in a Petri Dish, an 8-part series
How artificial intelligence, machine learning and emerging computational technologies are changing biomedical research and the future of health care
- Part 1 – Using machines to personalize patient care. Artificial intelligence and other computational techniques are aiding scientists and physicians in their quest to prescribe or create treatments for individuals rather than populations.
- Part 2 – Objective omics. Although the hypothesis is a core concept in science, unbiased omics methods may reduce attachments to incorrect hypotheses that can reduce impartiality and slow progress.
- Part 3 – Coding clinic. Rapidly evolving computational tools may unlock vast archives of untapped clinical information—and help solve complex challenges confronting health care providers.
- Part 4 – Scripting their own futures. At Sanford Burnham Prebys Graduate School of Biomedical Sciences, students embrace computational methods to enhance their research careers.
- Part 5 – Dodging AI and computational biology dangers. Sanford Burnham Prebys scientists say that understanding the potential pitfalls of using AI and other computational tools to guide biomedical research helps maximize benefits while minimizing concerns.
- Part 6 – Mapping the human body to better treat disease. Scientists synthesize supersized sets of biological and clinical data to make discoveries and find promising treatments.
- Part 7 – Simulating science or science fiction? By harnessing artificial intelligence and modern computing, scientists are simulating more complex biological, clinical and public health phenomena to accelerate discovery.
- Part 8 – Acceleration by automation. Increases in the scale and pace of research and drug discovery are being made possible by robotic automation of time-consuming tasks that must be repeated with exhaustive exactness.