At Sanford Burnham Prebys Graduate School of Biomedical Sciences, students embrace computational methods to enhance their research careers
Although not every scientist-in-training will need to be an ace programmer, the next generation of scientists will need to take advantage of advances in artificial intelligence (AI) and computing that are shaping biomedical research. Scientists who understand how to best process, store, access and employ algorithms to analyze ever-increasing amounts of information will help lead the data revolution rather than follow in its wake.
“I think the way to do biology is very different from just a decade or so ago,” says Kevin Yip, PhD, a professor in the Cancer Genome and Epigenetics Program at Sanford Burnham Prebys and the director of the Bioinformatics Shared Resource. “Looking back, I could not have imagined playing much of a role as a data scientist, and now I see that my peers and I are at the core of the whole discovery process.”
In 2017, bioinformatics experts suggested in Genome Biology that graduate education programs should focus on teaching computational biology to all learners rather than just those with a special interest in programming or data science. The authors noted that the changing nature of the life sciences required researchers to respond in kind. Teams of scientists must be able to formulate algorithms to keep pace and detect new discoveries obscured within oceans of data too vast to parse with prior methods.
“I think most people now would agree that data science and the use of computational methods—AI included—are indispensable in biology,” says Yip. “To use these approaches to the greatest effect, computational biologists and bench laboratory scientists need to be trained to speak a common language.”
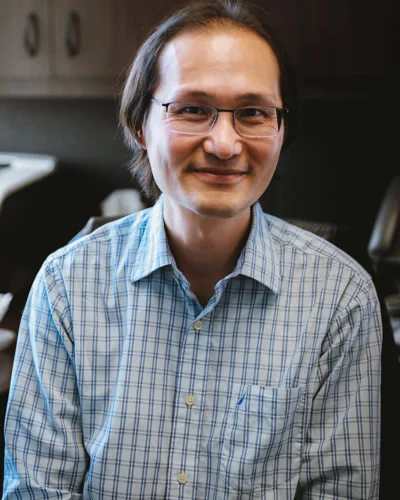
Kevin Yip, PhD, is a professor in the Cancer Genome and Epigenetics Program at Sanford Burnham Prebys.
When Yip joined Sanford Burnham Prebys in 2022, he was tasked with directing a course on computational biology for the Institute’s Graduate School of Biomedical Sciences.
“We believe that the new generation of graduate students needs to have the ability to understand what algorithms are and how they work, rather than just treating those tools as black boxes,” says Yip. “They may not be able to invent new algorithms right out of the course, but they’ll be better equipped to participate in collaborative projects.”
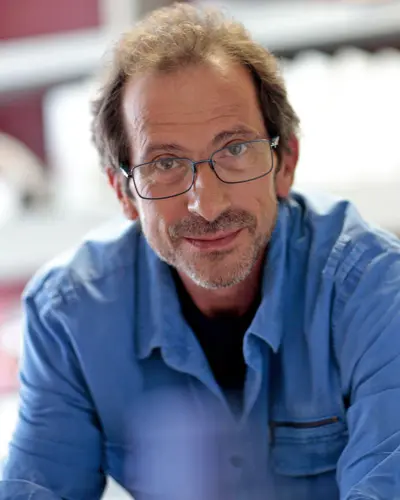
Andrei Osterman, PhD, is a professor in the Immunity and Pathogenesis
Program at Sanford Burnham Prebys.
Yip’s work developing the course has been well-received by graduate students based on their evaluations of the class.
“I loved the computational biology course,” says Katya Marchetti, a second-year PhD student in the lab of Karen Ocorr, PhD, and the recipient of an Association for Women in Science scholarship.
“It was so helpful to learn skills that I could immediately see incorporating into my own research. I’m so glad I had this course. I know for a fact that I will need this knowledge and experience to be successful in whatever comes after my PhD. The people who have these skills objectively do better in postdoctoral fellowships or in the biotechnology industry.”
Yip and his fellow faculty members in the graduate school see an opportunity to further expand their approach to computational biology and data science topics.
“In the current course, students learn to use computational methods to analyze transcriptomics data,” says Andrei Osterman, PhD, vice dean and associate dean of Curriculum for the Graduate School and a professor in the Immunity and Pathogenesis Program at Sanford Burnham Prebys. “This is very useful hands-on training, but not advanced enough for some students.”
“We are seeing students with a computer science background coming into our graduate program,” notes Yip. “We are thinking about adding a new elective course for students who want to go beyond what our current class is offering.”
Graduate education is quickly evolving at Sanford Burnham Prebys and throughout the biomedical research community to match the demands of an era defined by effectively integrating computation and biology.
“Mutual understanding among data scientists and biologists is very important for where research is heading,” says Yip. “We will keep improving our training to set our students up for success.”
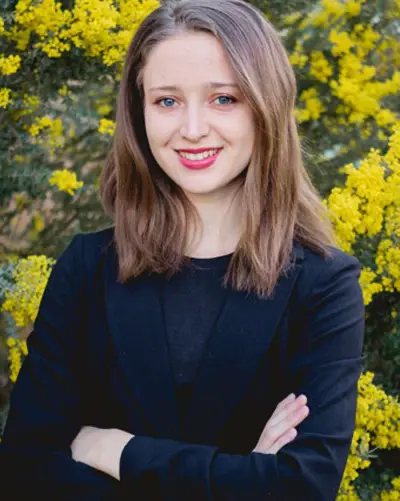
Katya Marchetti is a second-year PhD student at Sanford Burnham Prebys.
Programming in a Petri Dish, an 8-part series
How artificial intelligence, machine learning and emerging computational technologies are changing biomedical research and the future of health care
- Part 1 – Using machines to personalize patient care. Artificial intelligence and other computational techniques are aiding scientists and physicians in their quest to prescribe or create treatments for individuals rather than populations.
- Part 2 – Objective omics. Although the hypothesis is a core concept in science, unbiased omics methods may reduce attachments to incorrect hypotheses that can reduce impartiality and slow progress.
- Part 3 – Coding clinic. Rapidly evolving computational tools may unlock vast archives of untapped clinical information—and help solve complex challenges confronting health care providers.
- Part 4 – Scripting their own futures. At Sanford Burnham Prebys Graduate School of Biomedical Sciences, students embrace computational methods to enhance their research careers.
- Part 5 – Dodging AI and computational biology dangers. Sanford Burnham Prebys scientists say that understanding the potential pitfalls of using AI and other computational tools to guide biomedical research helps maximize benefits while minimizing concerns.
- Part 6 – Mapping the human body to better treat disease. Scientists synthesize supersized sets of biological and clinical data to make discoveries and find promising treatments.
- Part 7 – Simulating science or science fiction? By harnessing artificial intelligence and modern computing, scientists are simulating more complex biological, clinical and public health phenomena to accelerate discovery.
- Part 8 – Acceleration by automation. Increases in the scale and pace of research and drug discovery are being made possible by robotic automation of time-consuming tasks that must be repeated with exhaustive exactness.