Although the hypothesis is a core concept in science, unbiased omics methods may reduce attachments to incorrect hypotheses that can reduce impartiality and slow progress
Biological techniques that study the entire landscape of a sample’s genes or proteins—genomics or proteomics, respectively—help scientists discover new results without becoming too narrowly focused on what they predicted would happen. Although some scientists pursuing studies with this wider lens have been accused of going on “fishing expeditions,” many researchers counter that they now are able to investigate their hypotheses without missing other important results.
“I am a major proponent of omics, and especially unbiased omics,” says Sanju Sinha, PhD, an assistant professor in the Cancer Molecular Therapeutics Program at Sanford Burnham Prebys. “If someone now doesn’t show me unbiased results, it deeply bothers me. If every experiment only shows results from one pathway, it’s concerning and increases my skepticism about the study.”
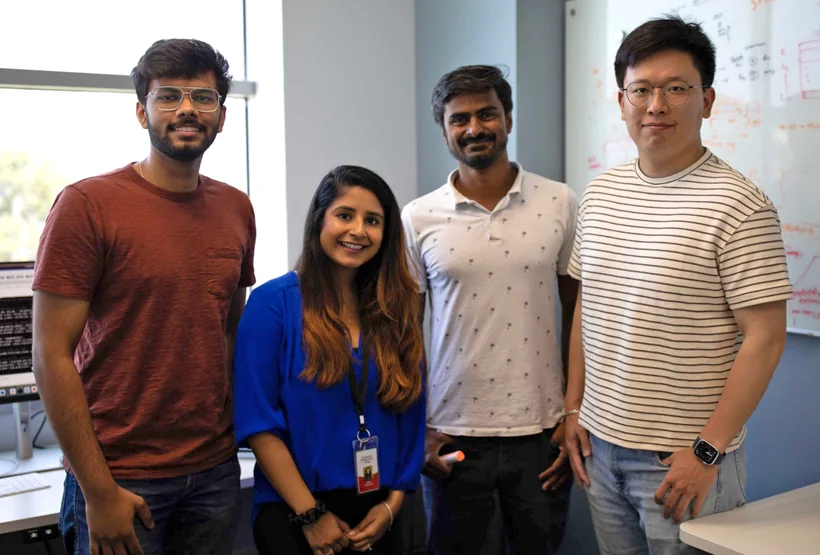
The Sinha Lab
An omics approach differs from traditional hypothesis-driven research in that it includes a comprehensive perspective about the phenomenon a scientist is studying and what might be causing it.
“Unbiased omics look at the global picture of how everything is changing,” explains Sinha. “If you’re looking at genetic factors, you present all 20,000 genes and how they change, rather than just one pathway and maybe 10 genes.”
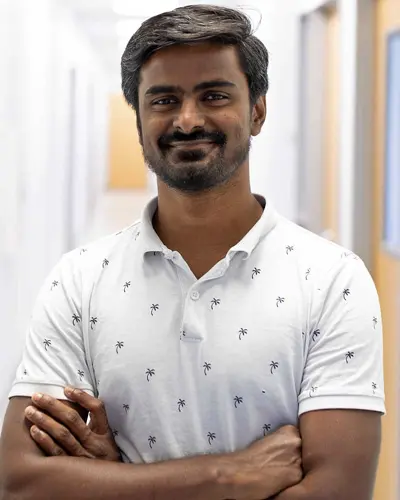
Sanju Sinha, PhD, is an assistant professor in the Cancer Molecular Therapeutics Program at Sanford Burnham Prebys.
This method reflects the dynamic nature of biomedical research.
“Biomedical research is currently experiencing a period of accelerating and metamorphic discoveries fueled by unparalleled technologies that generate enormous amounts of data that, in turn, spur and spawn avenues of new inquiry and questions previously unimagined,” says David A. Brenner, MD, president and CEO of Sanford Burnham Prebys.
“An effective and successful biomedical researcher in the 21st century requires input from different disciplines that previously were not part of standard practice or the scientific method.”
Sinha agrees. “People used to work in small silos. They could work on the same biological pathway for 30 years.” The new model, he said, is quickly shifting to more multidisciplinary, team-based science where experts from many fields collaborate to make the most of new technology and the rich data it can provide.
Some teams employing these omics approaches have been criticized for conducting aimless studies due to the lack of traditional hypotheses. Sinha is quick to defend against these claims.
“I don’t mind these so-called fishing expeditions. I like to say that there are only two kinds of science: applied science and not-yet-applied science. Fishing expeditions are valuable if the data is made available and other scientists can make discoveries with it for years to come.”
“We should remember that fishing expeditions in biomedical research have done a great service to humanity.”
The hypothesis is not an endangered species destined to be replaced by unbiased omics approaches. On the contrary, omics experiments can often be kick-starters that help scientists generate new hypotheses to explore.
A team of scientists at Sanford Burnham Prebys and their collaborators are using an omics technique called resistomics to develop a new class of antibiotics effective against a drug-resistant pathogen.
n a paper published on January 3, 2024 in Nature, a multi-institutional team including Andrei Osterman, PhD, a professor in the Immunity and Pathogenesis Program at Sanford Burnham Prebys, with colleagues at Roche—the Swiss-based pharmaceutical/healthcare company—and others, describe a novel class of small-molecule-tethered macrocyclic peptide (MCP) antibiotics with potent antibacterial activity against carbapenem-resistant Acinetobacter baumannii (CRAB).
The World Health Organization and the Centers for Disease Control and Prevention have both categorized multidrug-resistant A. baumannii as a top-priority pathogen and public health threat.
In the study, Osterman and colleagues applied an experimental evolution approach to help identify the drug target (the LPS transporter complex) of a new class of antibiotics—a macrocyclic peptide called Zosurabalpin—and elucidate the dynamics and mechanisms of acquired drug resistance in four distinct strains of A. baumannii.
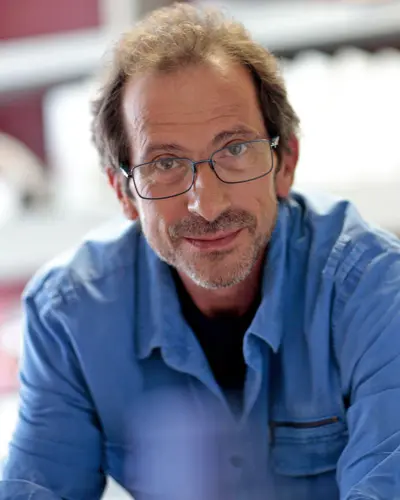
Andrei Osterman, PhD, is a professor in the Immunity and Pathogenesis program at Sanford Burnham Prebys.
They used an integrative workflow that employs continuous bacterial culturing in an “evolution machine” (morbidostat) followed by time-resolved, whole-genome sequencing and bioinformatics analysis to map resistance-inducing mutations.
“This comprehensive mapping of the drug-resistance landscape yields valuable insights for a variety of practical applications,” says Osterman, “from therapy optimization via genomics-based assessment of drug resistance/susceptibility of bacterial pathogens to a rational development of novel drugs with minimized resistibility potential.”
Programming in a Petri Dish, an 8-part series
How artificial intelligence, machine learning and emerging computational technologies are changing biomedical research and the future of health care
- Part 1 – Using machines to personalize patient care. Artificial intelligence and other computational techniques are aiding scientists and physicians in their quest to prescribe or create treatments for individuals rather than populations.
- Part 2 – Objective omics. Although the hypothesis is a core concept in science, unbiased omics methods may reduce attachments to incorrect hypotheses that can reduce impartiality and slow progress.
- Part 3 – Coding clinic. Rapidly evolving computational tools may unlock vast archives of untapped clinical information—and help solve complex challenges confronting health care providers.
- Part 4 – Scripting their own futures. At Sanford Burnham Prebys Graduate School of Biomedical Sciences, students embrace computational methods to enhance their research careers.
- Part 5 – Dodging AI and computational biology dangers. Sanford Burnham Prebys scientists say that understanding the potential pitfalls of using AI and other computational tools to guide biomedical research helps maximize benefits while minimizing concerns.
- Part 6 – Mapping the human body to better treat disease. Scientists synthesize supersized sets of biological and clinical data to make discoveries and find promising treatments.
- Part 7 – Simulating science or science fiction? By harnessing artificial intelligence and modern computing, scientists are simulating more complex biological, clinical and public health phenomena to accelerate discovery.
- Part 8 – Automation accelerating research.