Increases in the scale and pace of research and drug discovery are being made possible by robotic automation of time-consuming tasks that must be repeated with exhaustive exactness.
Humans have long been fascinated by automata, objects that can or appear to move and act of their own volition. From the golems of Jewish folklore to Pinocchio and Frankenstein’s Creature—among the subjects of many other tales—storytellers have long explored the potential consequences of creating beings that range from obedient robots to sentient saboteurs.
While the power of our imagination preceded the available technology for such feats of automation, many scientists and engineers throughout history succeeded in creating automata that were as amusing as they were examples of technical mastery. Three doll automata made by inventor Pierre Jaquet-Droz traveled around the world to delight kings and emperors by writing, drawing and playing music, and they now fascinate visitors to the Musée d’Art et d’Histoire of Neuchâtel, Switzerland.
While these more whimsical machinations can be found in collections from the House on the Rock in Spring Green, Wis., to the Hermitage Museum in Saint Petersburg, Russia, applications in certain forms of labor have made it so more modern automation is located in factories and workshops. There is no comparing the level of automation at research institutions to that of many manufacturing facilities more than 110 years since the introduction of the assembly line, nor should there be given the differing aims. However, the mechanization of certain tasks in the scientific process has been critical to increasing the accessibility of the latest biomedical research techniques and making current drug discovery methods possible.
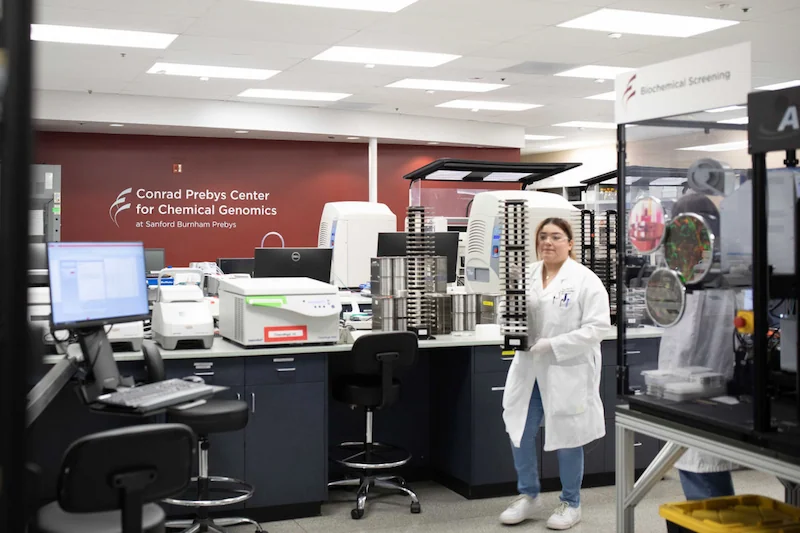
As a premier drug discovery center, the Conrad Prebys Center for Chemical Genomics team is well-versed in using automation to enable the testing of hundreds of thousands of chemicals to find new potential medicines.
“Genomic sequencing has become a very important procedure for experiments in many labs,” says Ian Pass, PhD, director of High-Throughput Screening at the Conrad Prebys Center for Chemical Genomics (Prebys Center) at Sanford Burnham Prebys. “Looking back just 20-30 years, the first sequenced human genome required the building of a robust international infrastructure and more than 12 years of active research. Now, with how we’ve refined and automated the process, I could probably have my genome sampled and sequenced in an afternoon.”
While many tasks in academic research labs require hands-on manipulation of pipettes, petri dishes, chemical reagents and other tools of the trade, automation has been a major factor enabling omics and other methods that process and sequence hundreds or thousands of samples to capture incredible amounts of information in a single experiment. Many of these sophisticated experiments would be simply too labor-intensive and expensive to conduct by hand.
Where some of the automation of yore would play a tune, enact a puppet show or tell a vague fortune upon inserting a coin, scientists now prepare samples for instruments equipped with advanced robotics, precise fluid handling technologies, cameras and integrated data analysis capabilities. Automation in liquid handling has enabled one of the biggest steps forward as it allows tests to be miniaturized. This not only results in major cost savings, but also it allows experiments to have many replicas, generating very high-quality, reliable data. These characteristics in data are a critical underpinning for ensuring the integrity of the scientific community’s findings and maintaining the public’s trust.
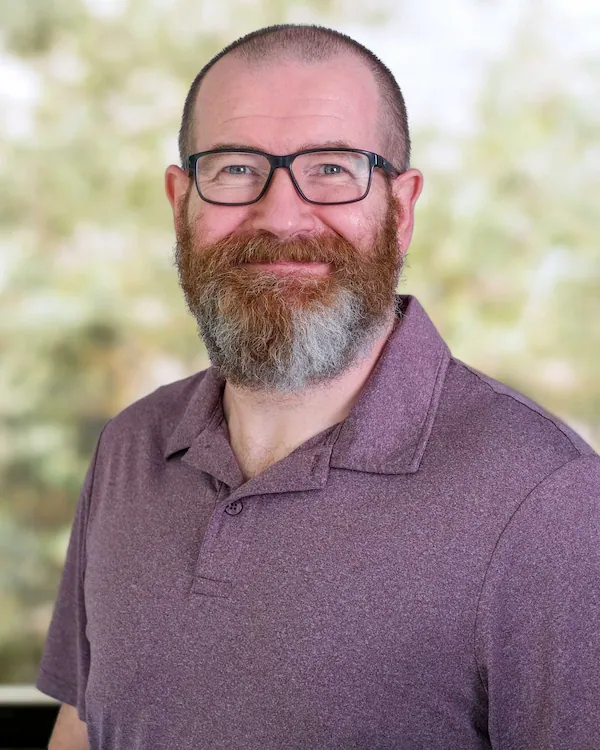
Ian Pass, PhD, is the director of High-Throughput Screening at the Conrad Prebys Center for Chemical Genomics.
“At their simplest, many robotic platforms amount to one or more arms that have a grip that can be programmed to move objects around,” explains Pass. “If a task needs to be repeated just a few times, then it probably isn’t worth the effort to deploy a robot. But, once that step needs to be repeated thousands of times at precise intervals, and handled the exact same way each time, then miniaturization and automation are the answers.”
As a premier drug discovery center, the Prebys Center team is well-versed in using automation to enable the testing of hundreds of thousands of chemicals to find new potential medicines. The center installed its first robotics platform, affectionately called “big yellow,” in the late 2000s to enable what is known as ultra-high-throughput screening (uHTS). Between 2009 and 2014, this robot was the workhorse for completing over 100 uHTS of a large chemical library. It generated tens of millions of data points as part of an initiative funded by the National Institutes of Health (NIH) called the Molecular Libraries Program that involved more than 50 research institutions across the US. The output of the program was the identification of hundreds of chemical probes that have been used to accelerate drug discovery and launch the field of chemical biology.
“Without automation, we simply couldn’t have done this,” says Pass. “If we were doing it manually, one experiment at a time, we’d still be on the first screen.”
Over the past 10 years the Center has shifted focus from discovering chemical probes to discovering drugs. Fortunately, much of the process is the same, but the scale of the experiments is even bigger, with screens of over 750,000 chemicals. To screen such large libraries, highly miniaturized arrays are used in which 1536 tests are conducted in parallel. Experiments are miniaturized to such an extent that hand pipetting is not possible and acoustic dispensing (i.e. sound waves) are used to precisely move the tiny amounts of liquid in a touchless, tipless automated process. In this way, more than 250,000 tests can be accomplished in a single day, allowing chemicals that bind to the drug target to be efficiently identified. Once the Prebys Center team identifies compounds that bind, these prototype drugs are then improved by the medicinal chemistry team, ultimately generating drugs with properties suitable for advancing to phase I clinical trials in humans.
Within the last year, the Prebys Center has retired “big yellow” and replaced it with three acoustic dispensing enabled uHTS robotic systems using 1536 well high-density arrays that can run fully independently.
“We used to use big yellow for just uHTP library screening, but now, with the new line up of robots, we use them for everything in the lab we can,” notes Pass. “It has really changed how we use automation to support and accelerate our science. Having multiple systems allows us to run simultaneous experiments and avoid scheduling conflicts. It also allows us to stay operational if one of the systems requires maintenance.”
One of the many drug discovery projects at the Prebys Center focuses on the national epidemic of opioid addiction. In 2021, fentanyl and other synthetic opioids accounted for nearly 71,000 of 107,000 fatal drug overdoses in the U.S. By comparison, in 1999 drug-involved overdose deaths totaled less than 20,000 among all ages and genders.
Like other addictive substances, opioids are intimately related to the brain’s dopamine-based reward system. Dopamine is a neurotransmitter that serves critical roles in memory, movement, mood and attention. Michael Jackson, PhD, senior vice president of Drug Discovery and Development at the Prebys Center and co-principal investigator Lawrence Barak, MD, PhD, at Duke University, have been developing a completely new class of drugs that works by targeting a receptor on neurons called neurotensin 1 receptor or NTSR1, that regulates dopamine release.
The researchers received a $6.3 million award from NIH and the National Institute on Drug Abuse (NIDA) in 2023 to advance their addiction drug candidate, called SBI-810, to the clinic. SBI-810 is an improved version of SBI-533, which previously had been shown to modulate NTSR1 signaling and demonstrated robust efficacy in mouse models of addiction without adverse side effects.
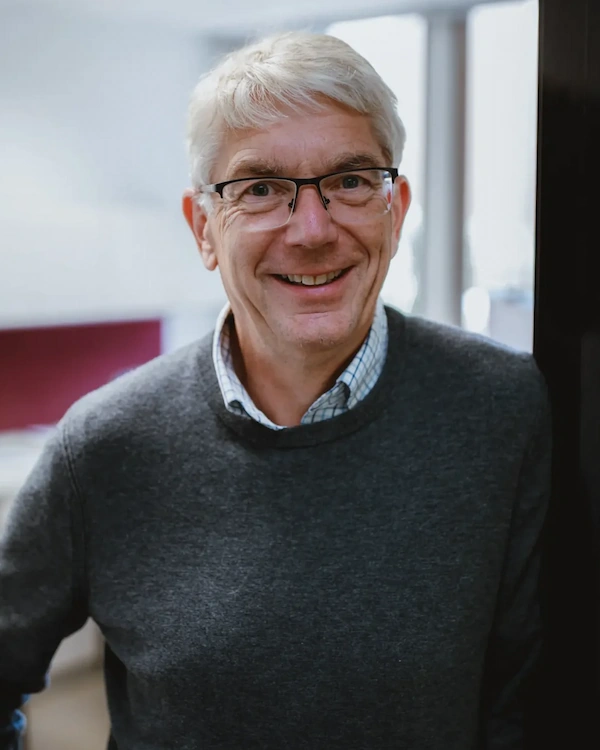
Michael Jackson, PhD, is the senior vice president of Drug Discovery and Development at the Conrad Prebys Center for Chemical Genomics.
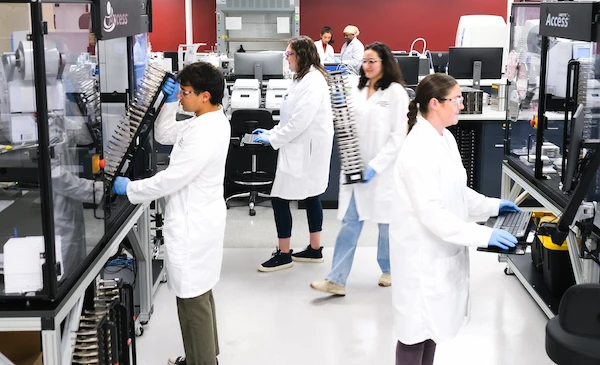
The funding from the NIH and NIDA will be used to complete preclinical studies and initiate a Phase 1 clinical trial to evaluate safety in humans.
“The novel mechanism of action and broad efficacy of SBI-810 in preclinical models hold the promise of a truly new, first-in-class treatment for patients affected by addictive behaviors,” says Jackson.
Programming in a Petri Dish, an 8-part series
How artificial intelligence, machine learning and emerging computational technologies are changing biomedical research and the future of health care
- Part 1 – Using machines to personalize patient care. Artificial intelligence and other computational techniques are aiding scientists and physicians in their quest to prescribe or create treatments for individuals rather than populations.
- Part 2 – Objective omics. Although the hypothesis is a core concept in science, unbiased omics methods may reduce attachments to incorrect hypotheses that can reduce impartiality and slow progress.
- Part 3 – Coding clinic. Rapidly evolving computational tools may unlock vast archives of untapped clinical information—and help solve complex challenges confronting health care providers.
- Part 4 – Scripting their own futures. At Sanford Burnham Prebys Graduate School of Biomedical Sciences, students embrace computational methods to enhance their research careers.
- Part 5 – Dodging AI and computational biology dangers. Sanford Burnham Prebys scientists say that understanding the potential pitfalls of using AI and other computational tools to guide biomedical research helps maximize benefits while minimizing concerns.
- Part 6 – Mapping the human body to better treat disease. Scientists synthesize supersized sets of biological and clinical data to make discoveries and find promising treatments.
- Part 7 – Simulating science or science fiction? By harnessing artificial intelligence and modern computing, scientists are simulating more complex biological, clinical and public health phenomena to accelerate discovery.
- Part 8 – Acceleration by automation. Increases in the scale and pace of research and drug discovery are being made possible by robotic automation of time-consuming tasks that must be repeated with exhaustive exactness.